In today’s digital age, businesses are increasingly focused on optimizing the customer journey to enhance satisfaction, loyalty, and profitability. Customer journey optimization involves understanding and improving every interaction a customer has with a brand, from initial awareness to post-purchase support. The advent of Artificial Intelligence (AI) and Machine Learning (ML) has revolutionized this field, providing new tools and insights that enable businesses to deliver personalized, efficient, and seamless experiences.
Brief Overview of Customer Journey Optimization
Customer experience managemeny is the practice of analyzing and refining the entire customer experience. This includes mapping out all touchpoints, identifying pain points, and implementing strategies to improve the overall journey. The goal is to create a smooth, cohesive experience that meets customer expectations and drives engagement.
Understanding AI and Machine Learning
Definitions and Basic Principles of AI and Machine Learning
Artificial Intelligence (AI): AI encompasses a broad range of technologies that enable machines to mimic human cognitive functions such as learning, problem-solving, and decision-making.
Machine Learning (ML): ML is a subset of AI that focuses on the development of algorithms that allow computers to learn from and make decisions based on data. ML models improve their performance over time as they are exposed to more data.
Key Differences Between AI and Machine Learning
While AI and ML are closely related, they are not synonymous. AI is the broader concept of machines being able to carry out tasks in a way that we would consider “smart.” ML, on the other hand, is a specific approach to achieving AI, characterized by the use of data and algorithms to train models to perform tasks autonomously.
Role of AI in Customer Journey Management
How AI Can Enhance Customer Journey Mapping
AI enhances customer journey mapping by analyzing vast amounts of data to identify patterns and insights that would be difficult for humans to detect. According to a study by McKinsey, companies that leverage AI in their customer journey mapping processes report a 10-15% increase in conversion rates and a 20-30% improvement in customer satisfaction scores.
Examples of AI Applications
- Chatbots and Virtual Assistants: These AI-powered tools provide instant support to customers, answering queries, resolving issues, and guiding them through the purchasing process. Gartner predicts that by 2025, 80% of customer interactions will be handled by AI-powered systems.
- Recommendation Systems: AI algorithms analyze customer data to suggest products or services that are likely to be of interest, enhancing the shopping experience and driving sales. Amazon reports that 35% of its revenue is generated by its recommendation engine.
- Sentiment Analysis: AI can analyze customer feedback and social media interactions to gauge customer sentiment, helping businesses address concerns and improve satisfaction. A survey by Forrester found that companies using AI for sentiment analysis saw a 25% reduction in customer churn.
Machine Learning in Customer Journey Management
Explanation of How Machine Learning Algorithms Work
Machine learning algorithms learn from data by identifying patterns and making predictions. They can be supervised (trained on labeled data), unsupervised (finding patterns in unlabeled data), or semi-supervised. These algorithms continuously improve as they process more data, making them highly effective for dynamic environments like customer journey management.
Use Cases of Machine Learning
- Predicting Customer Behavior: ML models can analyze past behavior to predict future actions, such as the likelihood of making a purchase or churning. According to a report by PwC, predictive analytics can increase sales by up to 10% and reduce churn by up to 20%.
- Segmenting Customers: ML can identify distinct customer segments based on behavior, preferences, and demographics, allowing for more targeted marketing efforts. Research by Accenture found that personalized marketing campaigns driven by ML can boost revenue by 5-15%.
- Personalizing Experiences: ML algorithms can tailor content, offers, and interactions to individual customers, enhancing their experience and increasing engagement. Epsilon’s research indicates that personalized experiences can result in a 20% increase in customer satisfaction.
Benefits of Using AI and Machine Learning
Improved Customer Insights and Data Analysis
AI and ML provide deeper insights into customer behavior by analyzing large datasets quickly and accurately. This helps businesses to understand customer needs and preferences better. A study by Deloitte found that companies using AI for customer insights reported a 33% improvement in customer understanding.
Enhanced Personalization and Customer Experience
AI and ML enable highly personalized interactions by leveraging customer data to tailor experiences to individual preferences, leading to higher satisfaction and loyalty. According to Salesforce, 76% of customers expect companies to understand their needs and expectations.
Increased Efficiency and Reduced Manual Effort
Automation powered by AI and ML reduces the need for manual intervention in various processes, such as customer support and marketing, resulting in increased efficiency and cost savings. McKinsey estimates that AI can automate 45% of activities that individuals are paid to perform, leading to significant cost reductions.
Real-Time Decision-Making Capabilities
AI and ML allow businesses to make data-driven decisions in real-time, responding quickly to changing customer behaviors and market conditions. A survey by Capgemini found that 77% of businesses believe that AI improves decision-making and efficiency.
Practical Applications and Examples
Case Studies of UAE Companies
- Noon: This e-commerce giant uses AI to personalize shopping experiences, recommend products, and optimize logistics. AI-driven analytics help Noon understand customer preferences and behavior, leading to more targeted marketing and improved customer satisfaction. Noon reports a 20% increase in sales due to AI-driven recommendations.
- Careem: The ride-hailing service leverages AI and ML to predict demand, optimize routes, and personalize user experiences. AI algorithms analyze user data to offer tailored promotions and improve service efficiency. Careem has seen a 15% reduction in wait times and a 10% increase in customer retention.
- Mashreq Bank: This financial institution employs AI to enhance customer service through chatbots and predictive analytics. AI helps Mashreq Bank to offer personalized financial advice and improve fraud detection. The bank reports a 30% increase in customer engagement and a 25% reduction in fraud.
Specific Examples
- Predictive Analytics for Customer Retention: AI models predict which customers are likely to churn and suggest retention strategies, such as targeted offers or personalized outreach. According to a study by Bain & Company, reducing churn by 5% can increase profitability by 25-95%.
- Dynamic Pricing Models: ML algorithms adjust prices in real-time based on demand, competition, and customer behavior, optimizing revenue and customer satisfaction. Companies using dynamic pricing report an average revenue increase of 10-20%.
- Automated Customer Support: AI-powered chatbots handle common customer queries, freeing up human agents to focus on more complex issues and improving response times. IBM reports that businesses using AI-powered customer support see a 30% reduction in customer service costs.
Challenges and Considerations
Potential Challenges in Implementing AI and Machine Learning
- Data Privacy Concerns: Collecting and analyzing customer data raises privacy issues. Businesses must ensure compliance with data protection regulations and maintain customer trust. According to Cisco, 84% of consumers care about data privacy, and 48% have switched companies over data privacy concerns.
- Need for Large Datasets: Effective AI and ML models require large amounts of high-quality data, which can be challenging to obtain and manage. A survey by O’Reilly found that 56% of organizations struggle with data quality issues.
Ethical Considerations and the Importance of Transparency
Businesses must address ethical issues, such as bias in AI algorithms, and ensure transparency in how AI and ML are used. This includes being clear with customers about data collection practices and the use of AI in decision-making. The World Economic Forum emphasizes that ethical AI practices are crucial for maintaining customer trust and avoiding potential legal issues.
Future Trends and Innovations
Emerging Trends in AI and Machine Learning
- AI-Driven Customer Journey Orchestration Platforms: These platforms use AI to automate and optimize every touchpoint in the customer journey, providing a seamless and personalized experience. Gartner predicts that by 2026, 60% of large enterprises will use AI-driven orchestration platforms to improve customer journeys.
- Sentiment Analysis Tools: Advanced AI tools analyze customer emotions and sentiments in real-time, enabling businesses to respond promptly and appropriately. MarketsandMarkets projects that the sentiment analysis market will grow from AED 11 billion in 2020 to AED 23 billion by 2025.
Innovations
- AI-Powered Predictive Analytics: Future AI tools will offer even more precise predictions of customer behavior, enabling proactive engagement and retention strategies. Research by Forrester suggests that predictive analytics can boost marketing ROI by 15-20%.
- Personalized Customer Experiences: AI will continue to evolve, offering deeper personalization through advanced data analysis and machine learning techniques. According to Accenture, 91% of consumers are more likely to shop with brands that provide relevant offers and recommendations.
Steps to Implement AI and Machine Learning in Customer Experience Management
Practical Steps for Businesses
- Assess Current Capabilities: Evaluate existing data, tools, and processes to identify areas where AI and ML can add value.
- Define Clear Objectives: Establish specific goals for AI and ML implementation, such as improving customer satisfaction or increasing efficiency.
- Invest in Data Quality and Integration: Ensure that data is accurate, complete, and integrated across systems to maximize the effectiveness of AI and ML models.
- Collaborate with Experts: Partner with AI and ML experts or vendors to leverage their knowledge and experience in implementing these technologies.
Conclusion
AI and machine learning are transforming the way businesses optimize customer journeys, offering unparalleled insights, personalization, and efficiency. By leveraging these technologies, companies can deliver superior customer experiences, drive engagement, and stay ahead of the competition. As AI and ML continue to evolve, the opportunities for enhancing customer journey management will only grow, making it essential for businesses to explore and embrace these innovations.
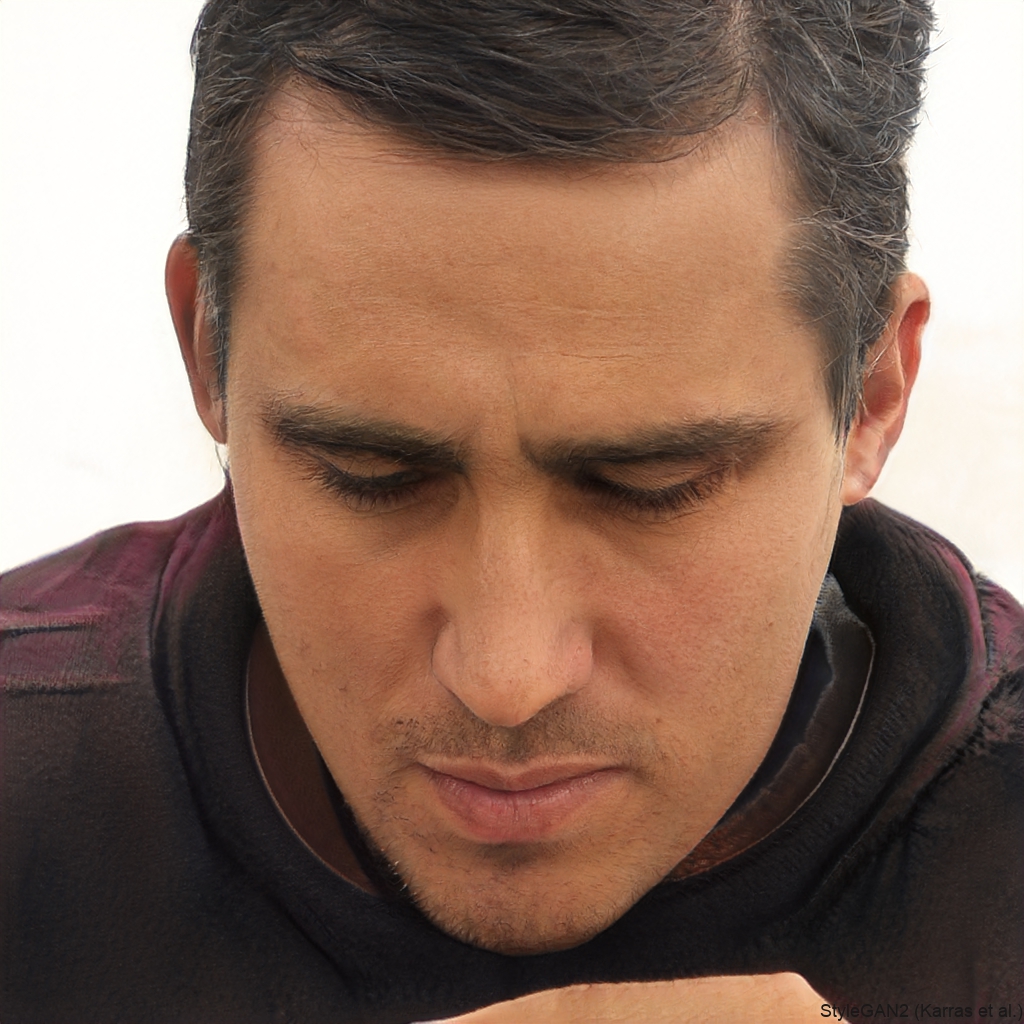
Surfer, foodie, record lover, Swiss design-head and ADC member. Performing at the sweet spot between simplicity and elegance to create not just a logo, but a feeling. Let’s chat.